When a recognition AI, realized by a deep neural network (DNN), faces the open world, it will inevitably deal with unexpected scenes. This includes unknown objects and unknown environments / domains that are out of distribution (OOD) with respect to the data encountered during training. DNNs typically suffer from significant degradation of performance when facing OOD objects or domain shifts. This can be seen as the main obstacle for the application of AI-driven perception in medicine, automated driving and open world robotics.
With the advent of vision transformers, large-scale foundation models and vision-language models, a new perspective towards significant progress on this set of problems arises. We invite researchers to submit their original or previously published works on methods and datasets that study and expand the capabilities of DNNs for recognition in an open world.
Join us on 27 November 2024 in shaping the future of AI-driven computer vision. We are looking forward to your innovative contributions.
Speakers
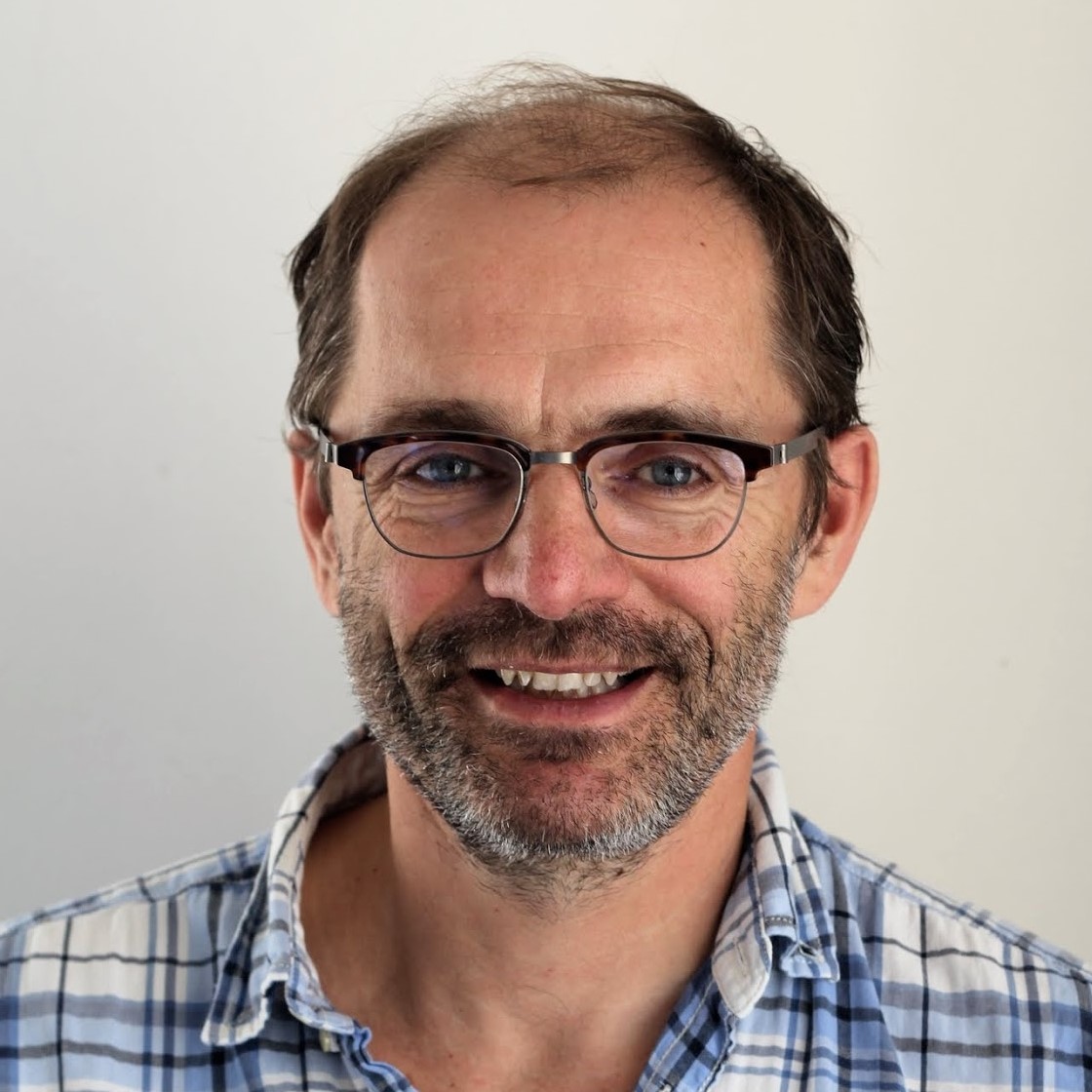
Jiří Matas
Head of the Visual Recognition Group at the Center for Machine Perception, CTU Prague.
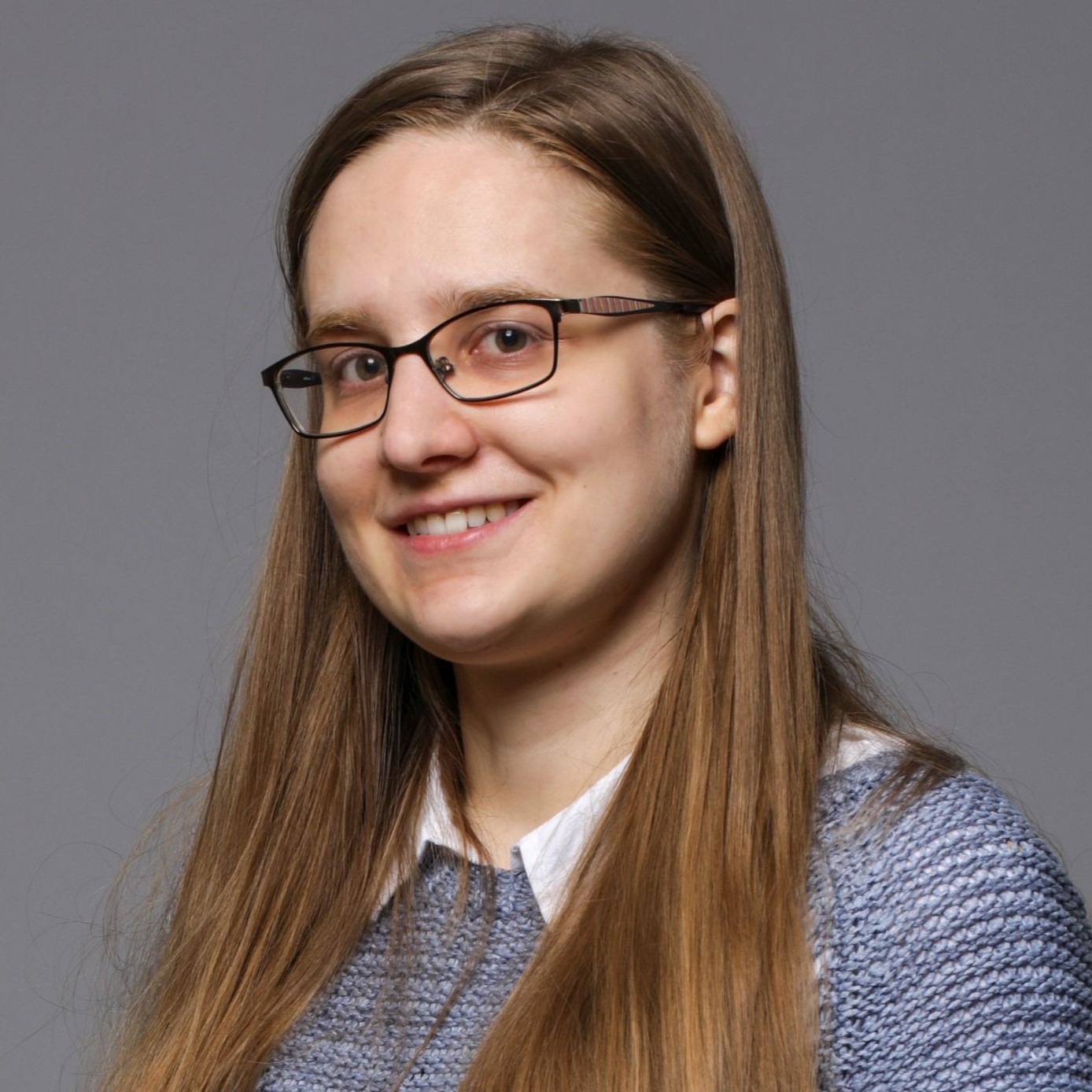
Petra Bevandić
Software developer and PostDoc working at the University of Bielefeld.
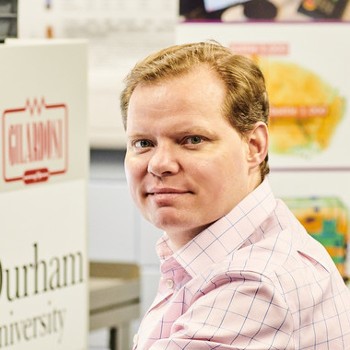
Toby Breckon
Professor in the department of computer science and engineering at Durham University.
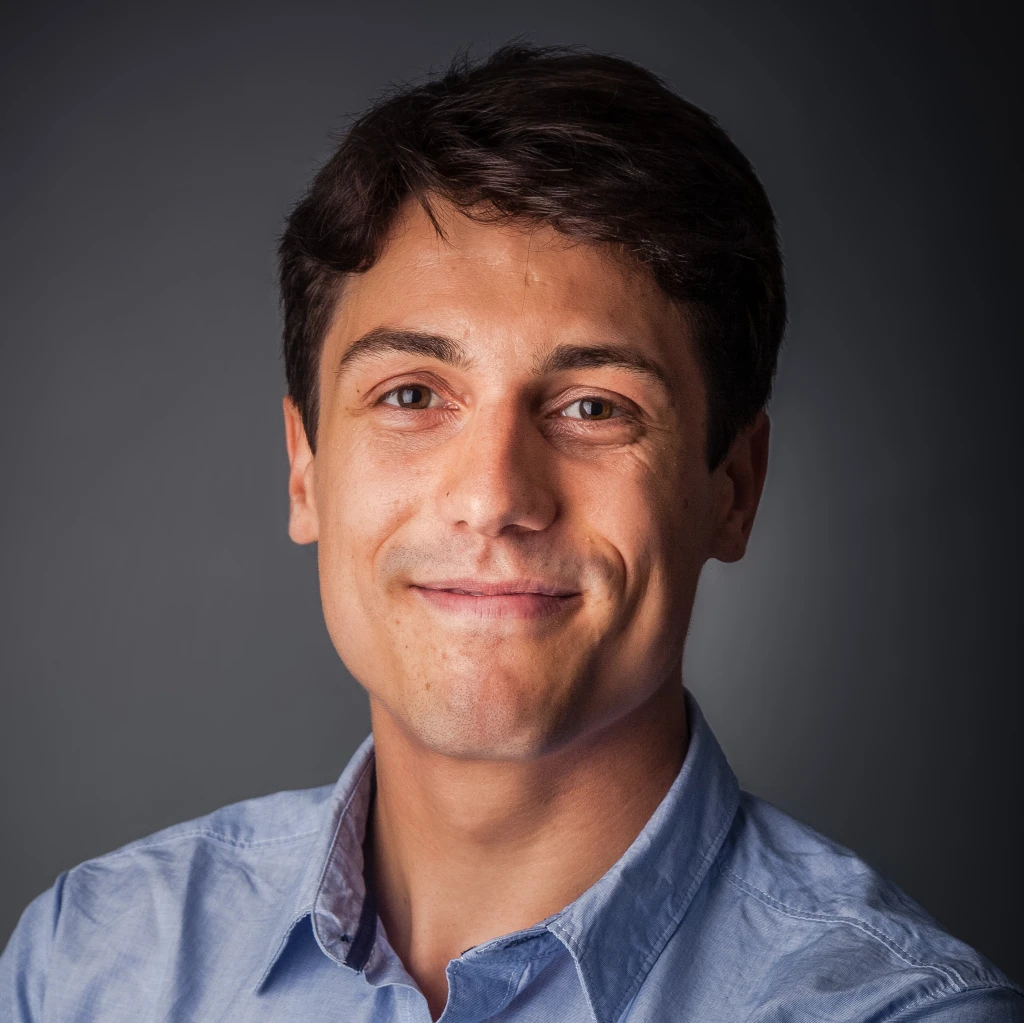
Robert Geirhos
Research Scientist at Google DeepMind.
Schedule
Workshop Date: 27 November 2024
Location: Scottish Exhibition Centre, Glasgow
Time | Topic |
---|---|
9:00 - 9:15 | Welcome |
9:15 - 9:50 | Invited Talk 1: "Robust Recognition with Image Decomposition" – Jiri Matas |
9:50 - 10:25 | Invited Talk 2: "Addressing Incompatible Taxonomies for Effective Multi-Dataset Training" – Petra Bevandic |
10:25 - 10:40 | Contributed Talk 1: 1) "UMAD: Unsupervised Mask-Level Anomaly Detection for Autonomous Driving" - Daniel Bogdoll, Noël Ollick, Tim Joseph, Svetlana Pavlitska, J. Marius Zöllner |
Short break | |
11:00 - 12:00 | Contributed Talks 2-5: 2) "Unsupervised Feature Orthogonalization for Learning Distortion-Invariant Representations" - Sebastian Doerrich, Francesco Di Salvo, Christian Ledig 3) "Hybrid Video Anomaly Detection for Autonomous Driving" - Daniel Bogdoll, Jan Imhof, Tim Joseph, Svetlana Pavlitska, J. Marius Zoellner 4) "Unsupervised Class Incremental Learning using Empty Classes" - Svenja Uhlemeyer, Julian Lienen, Youssef Shoeb, Eyke Hüllermeier, Hanno Gottschalk 5) "Uncertainty and Prediction Quality Estimation for Semantic Segmentation via Graph Neural Networks" - Edgar Heinert, Stephan Tilgner, Timo Palm, Matthias Rottmann |
12:00 - 12:35 | Invited Talk 3: "Object-wise Out of Distribution & Anomaly Detection Meets Real-World Complexity" – Toby Breckon |
Lunch break | |
14:05 – 14:50 | Contributed Talks 6-8: 6) "Impact of Surface Reflections in Maritime Obstacle Detection" - Samed YALÇIN, Hazım Kemal Ekenel 7) "Patchhealer: Counterfactual Image Segment Transplants with chest X-ray domain check" - Hakan Lane, Michal Valko, Grace Guo, Veda Sahaja Bandi, Nandini Lokesh Reddy, Stefan Kramer 8) "A Study on Unsupervised Domain Adaptation for Semantic Segmentation in the Era of Vision-Language Models" - Manuel Schwonberg, Claus Werner, Hanno Gottschalk, Carsten Meyer |
14:50 – 15:30 | Round table discussion: Petra Bevandić - Bielefeld University Toby Breckon - Durham University Fabian Hüger - CARIAD Jiří Matas - CTU Prague Moderator: Hanno Gottschalk - TU Berlin |
Short break | |
15:45 - 16:30 | Challenge Session: "OOD Tracking on Videos" |
16:30 – 17:05 | Invited Talk 4: "OOD Generalization with Generative Models" – Robert Geirhos |
17:05 - 17:30 | Best paper award, wrap up and closing remarks |
Challenge
For this workshop, we invite participants to tackle the challenge of Open-World Object Detection and Tracking. Develop AI models that excel in detecting and tracking objects in diverse and unpredictable environments, addressing out-of-distribution (OOD) objects and domain shifts.
Organizers
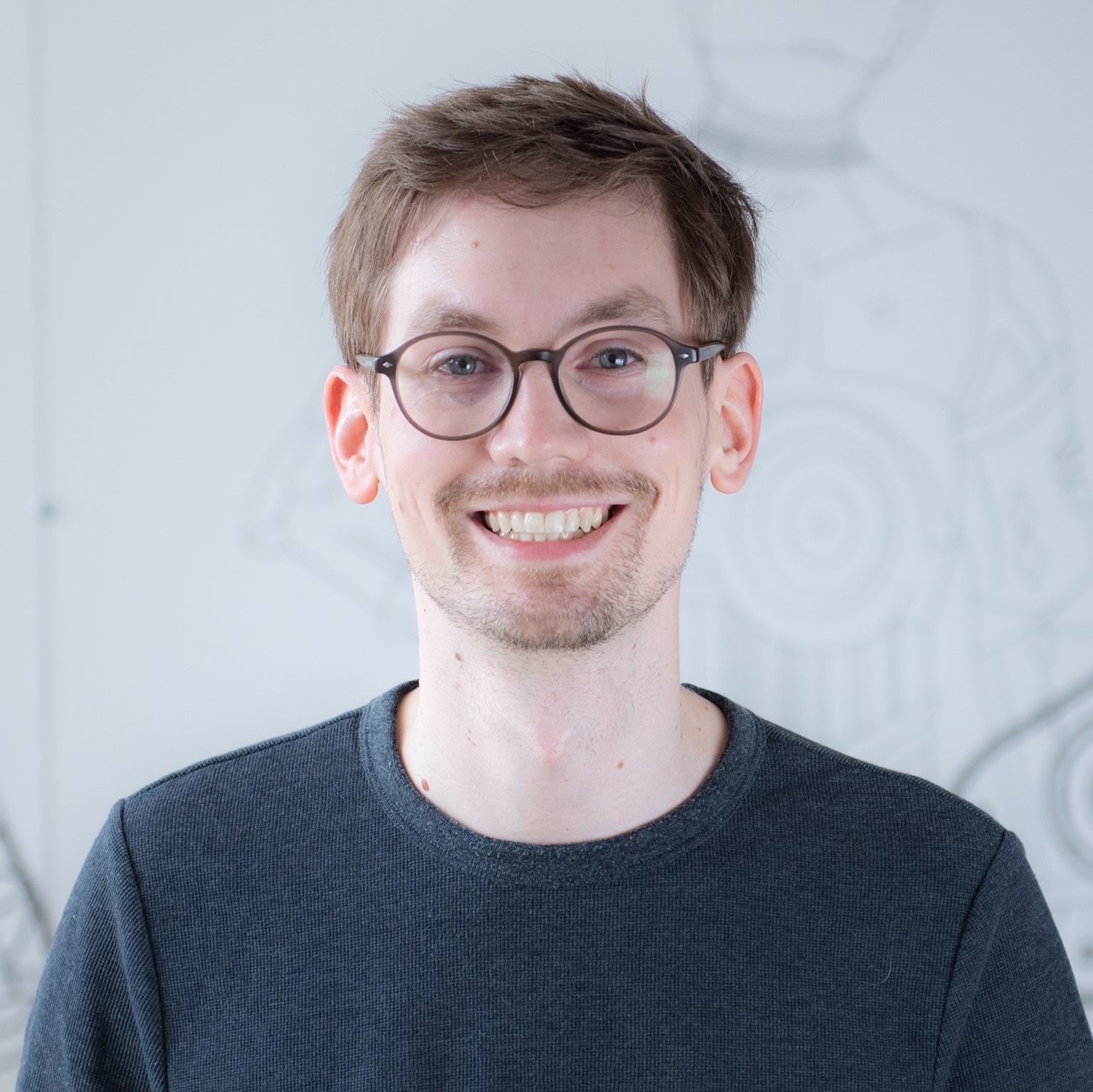
Hermann Blum
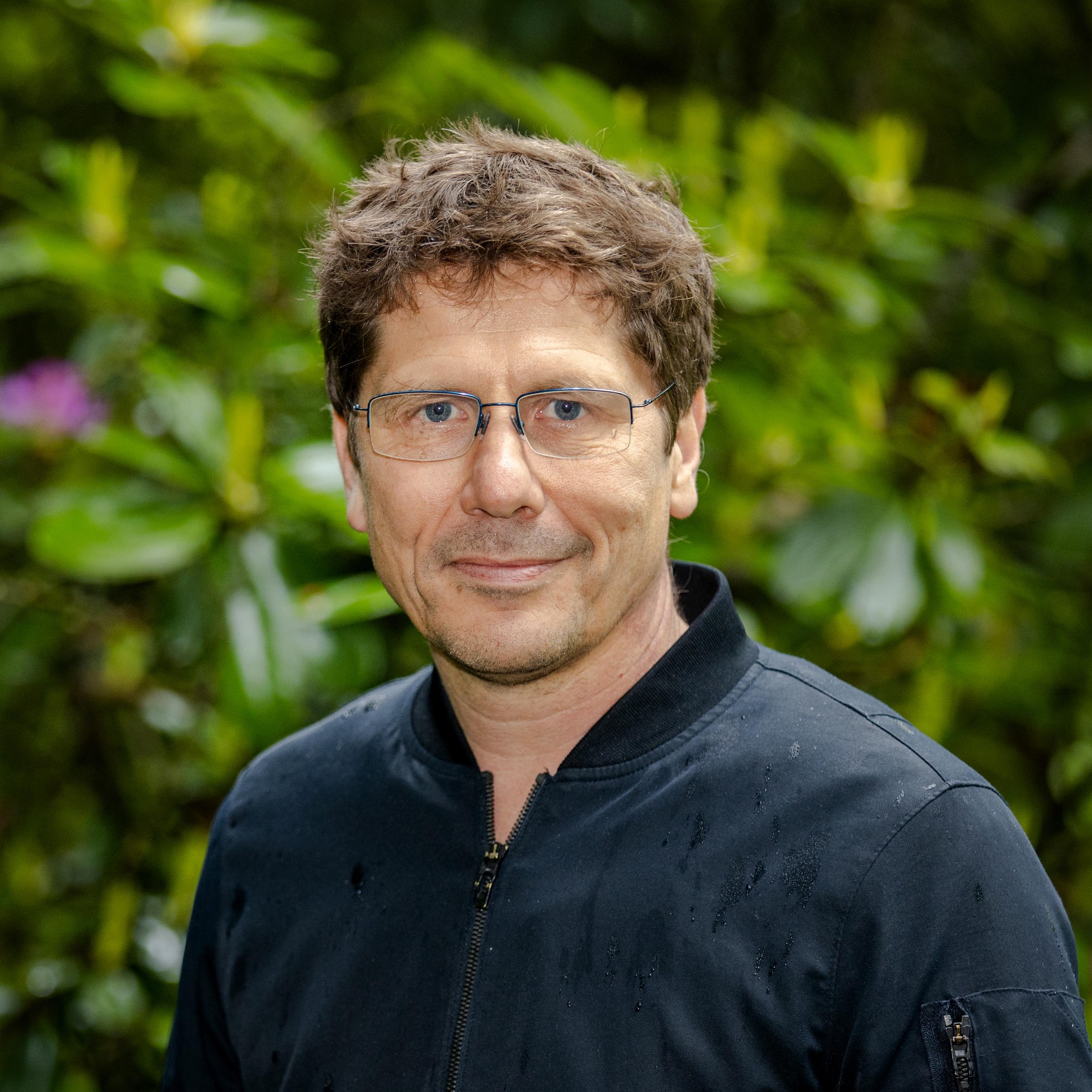
Hanno Gottschalk
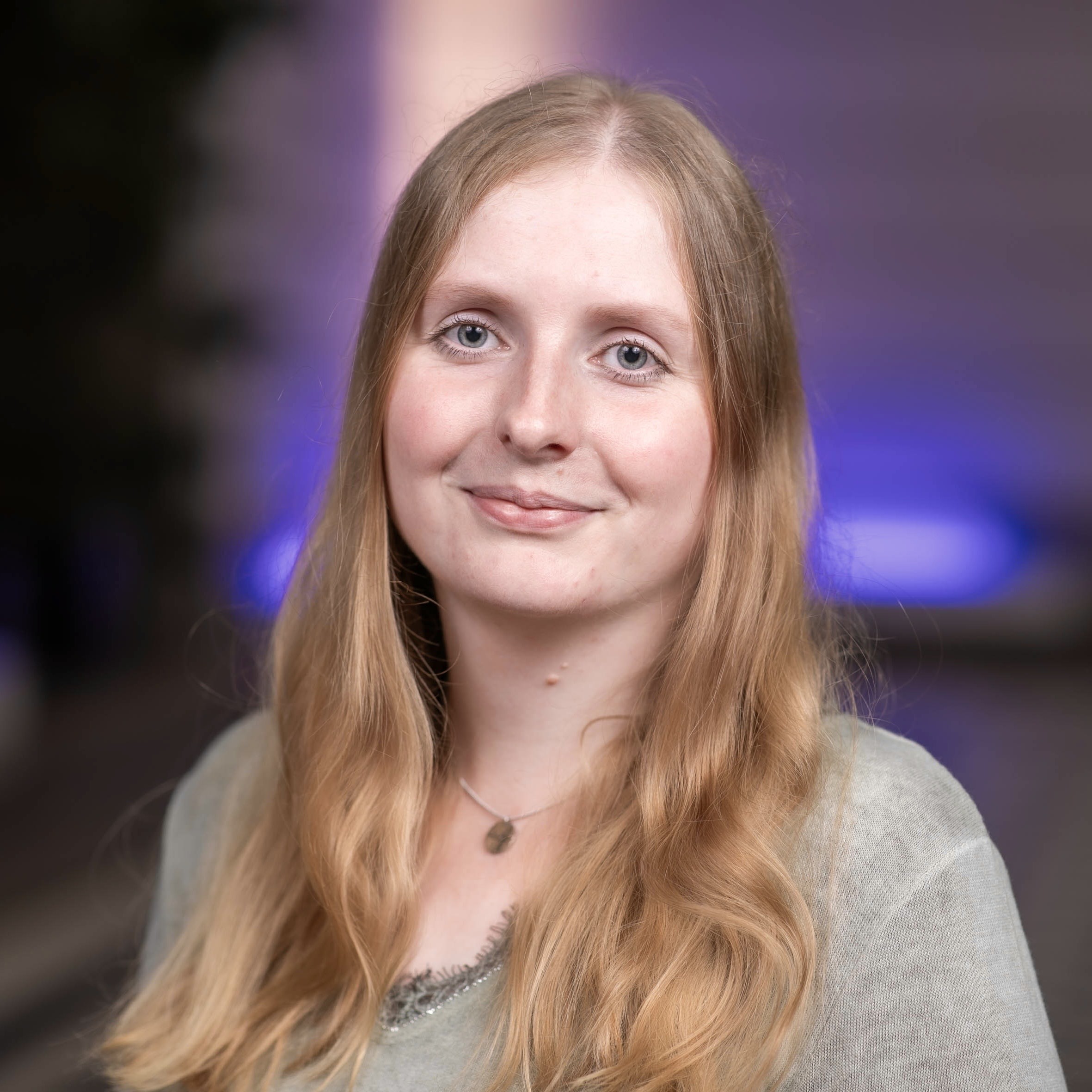
Kira Maag
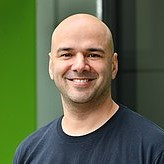
Matthias Rottmann
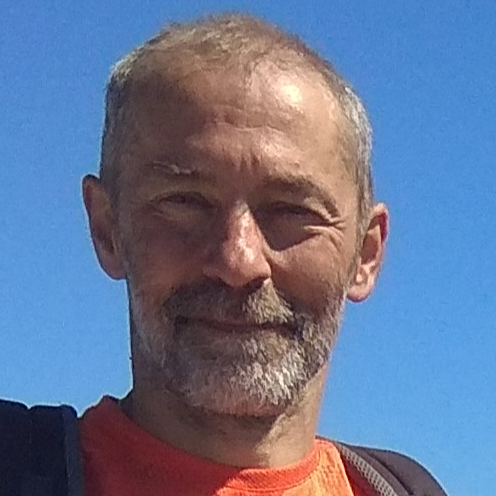
Siniša Šegvić
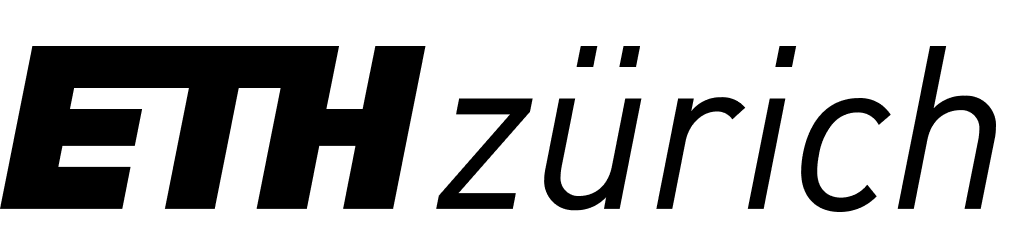
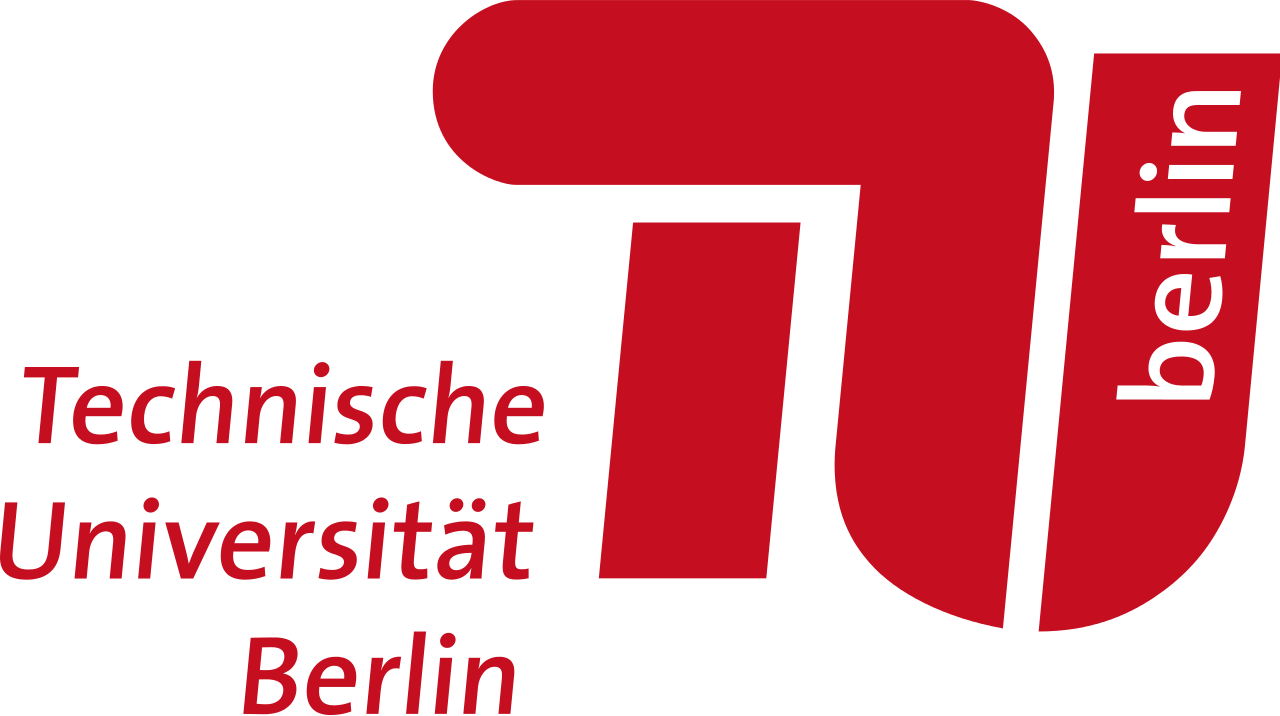
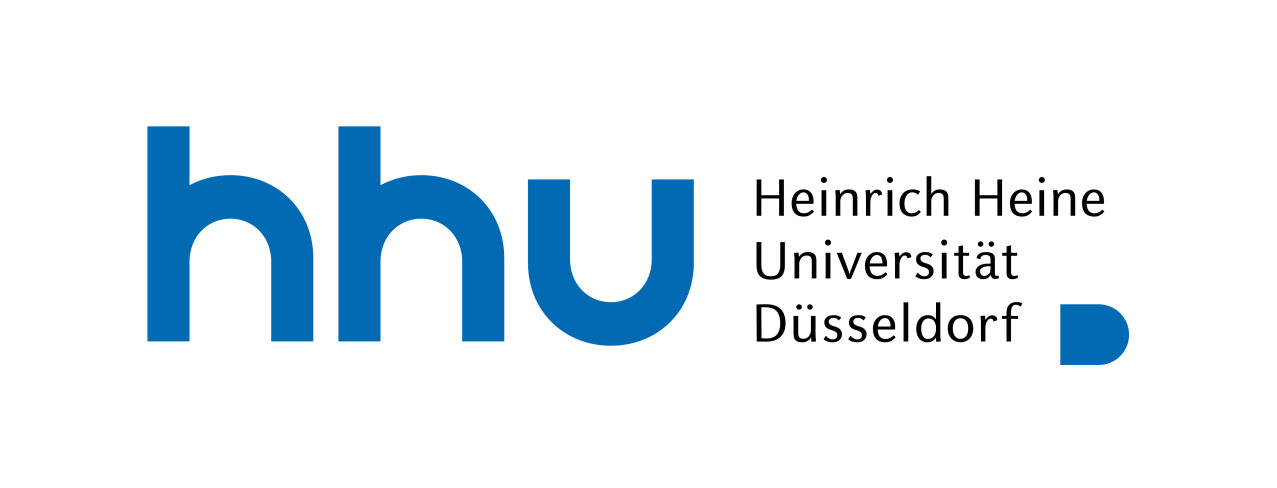
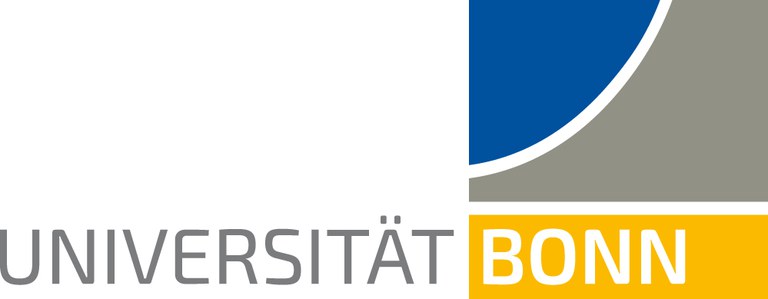
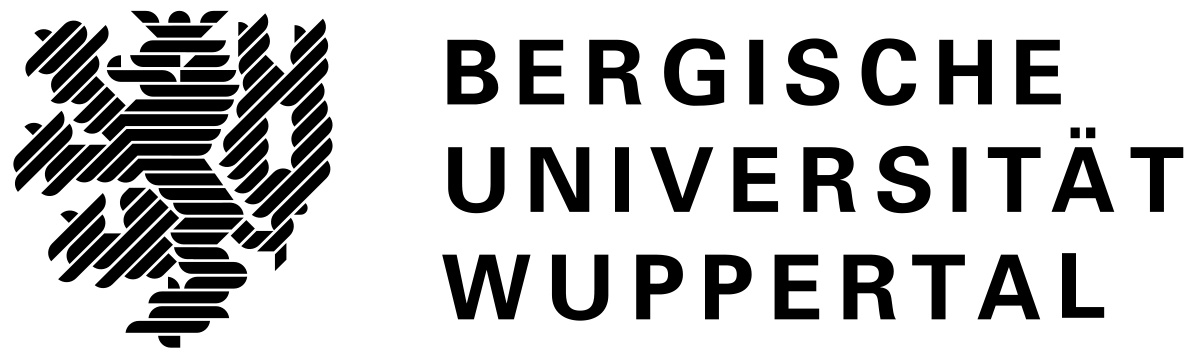
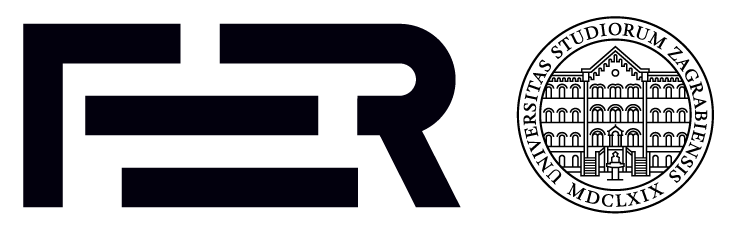
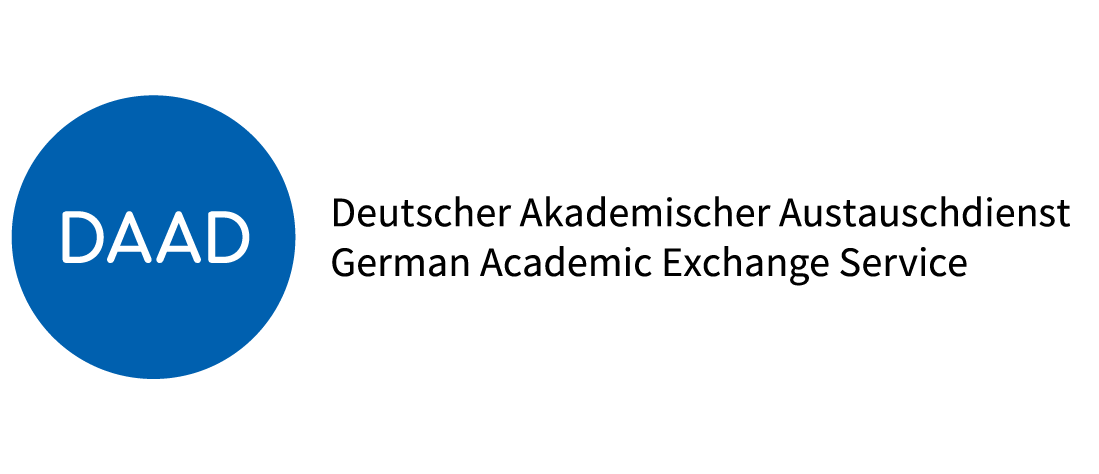
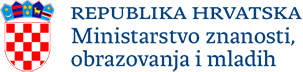
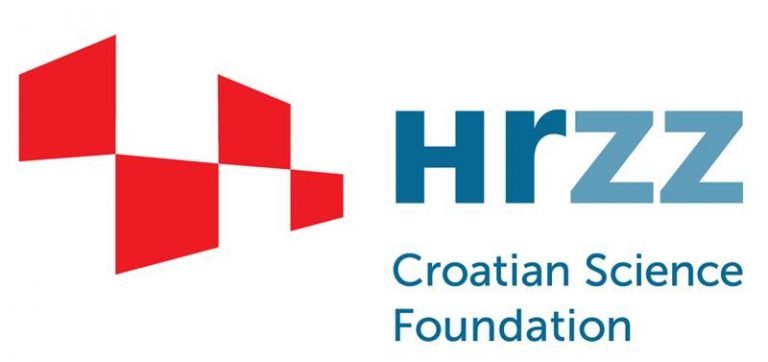